The dengue forecasting challenge: a step closer to better disease outbreak control and prevention?
London School of Hygiene & Tropical Medicine https://lshtm.ac.uk/themes/custom/lshtm/images/lshtm-logo-black.png Wednesday 13 November 2019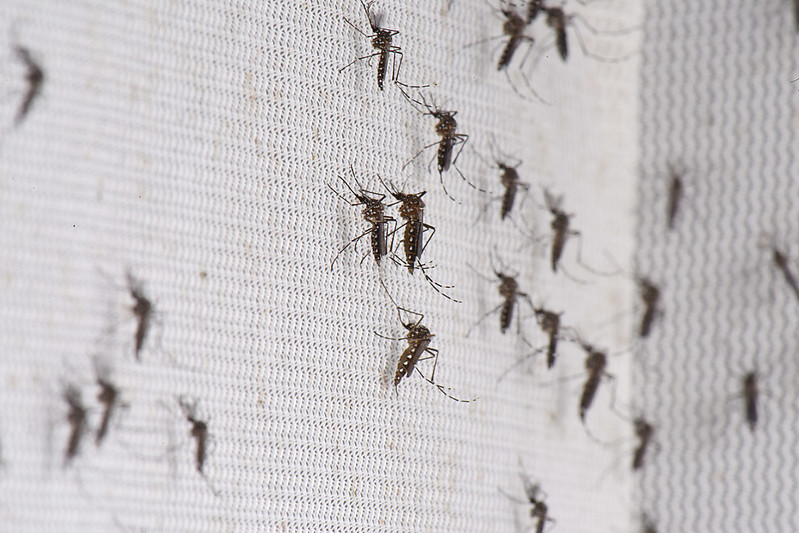
Although not a new concept in quantitative infectious disease research, outbreak forecasting has yet to be fully integrated into formal policy and decision-making processes in many parts of the globe. One reason for this is the mismatch between the forecasting goals set out by researchers and those considered useful by policy and decision-makers.
To align the efforts between the research and policy communities, multiple U.S. government agencies within the ‘Predict the Next Pandemic Initiative’, convened by the White House Office for Science and Technology, launched an open dengue forecasting challenge in 2015.
Dengue fever, a mosquito-borne viral infection, poses a significant public health challenge, with around half the world’s population estimated to be at risk. In areas where dengue is endemic, incidence follows seasonal transmission patterns punctuated every few years by much larger epidemics. Previous efforts have focused on predicting the risk of dengue outbreaks using climate forecasts.
However, this challenge was the first of its kind to combine multiple dengue forecasting models from multiple teams from around the globe, aiming to produce accurate forecasts of the timing and intensity of the peak dengue season in two important dengue endemic countries – Iquitos, Peru and San Juan, Puerto Rico.
Because epidemics in these places are currently unpredictable and of major consequence to the affected populations, the focus of the challenge was to forecast key features (e.g. size and timing of peak) for historical dengue seasons between 2005 and 2013 using only data from time periods prior to those seasons.
The specific outbreak forecasting goals were jointly created by U.S. health agencies, infectious disease modellers, and field epidemiologists who have worked in the study sites. The challenge involved 16 teams, two of which we led, and employed a wide variety of outbreak forecasting techniques (e.g. statistical models, mechanistic models) and innovative data sources (e.g., climate information, social media).
Published in the journal PNAS, the results of the challenge show that forecasting accuracy was highly variable across models, forecasting goals, and time at which forecasts were generated. Early-season forecasts, although more valuable to intervention planning, tend to be less accurate. Mid-to-late season forecasts, on the other hand, are more accurate and could be used for mobilising emergency resources in the short-term.
Although no single modelling approach consistently outperformed the others, certain characteristics led to higher forecasting accuracy. For example, statistical models, estimated from the historical data, outperformed mechanistic models, which used equations to represent biological processes.
So what has the challenge told us about strengthening disease outbreak forecasts?
First, it’s evident that most models have their particular strengths and weaknesses. In order to achieve maximum forecast accuracy, future research should focus on exploring ways that different models and approaches can be combined while compensating for each other’s limitations.
The challenge provided a standardised platform that allowed for extensive model comparison. Such an opportunity is rare and valuable for developing quantitative infectious disease research methodology. Dengue outbreak forecasters were able to test different approaches using real-world data and clearly demonstrate to public health policy and decision-makers the strengths and weakness of their “products”.
Second, while the challenge has not led to formal integration of routine dengue outbreak forecasting into local policy and decision processes, it did push the process forward. In fact, it can be considered a pilot project showcasing the relevance, feasibility, and applicability of outbreak forecasting techniques for dengue management, control and prevention.
Researchers, policy makers and public health practitioners must continue working together to ensure that we are applying the best science available for outbreak control and prevention, to target limited resources in a timely manner towards those communities most at risk.
Publications:
M.A. Johansson et al. An open challenge to advance probabilistic forecasting for dengue epidemics. PNAS. DOI: 10.1073/pnas.1909865116
LSHTM's short courses provide opportunities to study specialised topics across a broad range of public and global health fields. From AMR to vaccines, travel medicine to clinical trials, and modelling to malaria, refresh your skills and join one of our short courses today.